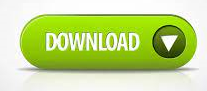
This is important in drug discovery because current drugs are limited in both structure and function. PCA can also be used to guide the design of chemical libraries.
Microsoft word add in for chemical structures full#
As such, the three-dimensional plot we show in this example retains 75 % of the variance from the full 20-dimensional dataset. PCA rotates these vectors onto a new set of orthogonal axes called principal components, in which the variance retained from the original data is maximized on each successive principal component.
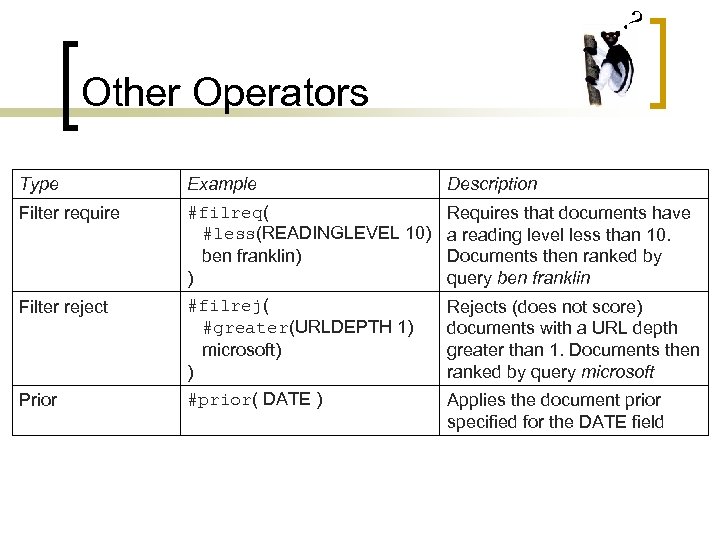
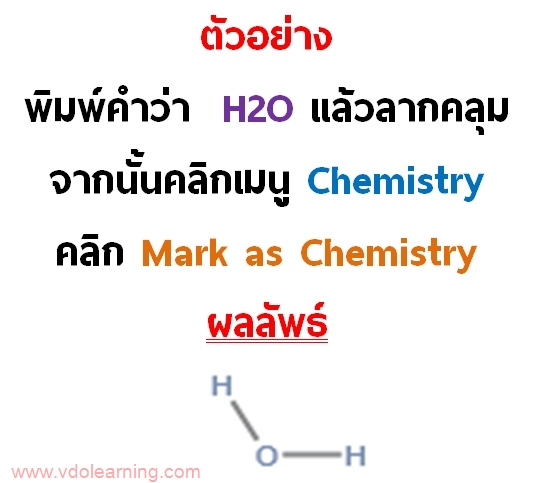
Each compound in our analysis is represented as a 20-dimensional vector defined by the structural and physicochemical parameters. Herein, we selected 20 structural and physicochemical parameters for analysis based on previously identified correlations of these parameters with oral bioavailability, cell permeability, solubility, and binding selectivity, as well as their ability to distinguish synthetic drugs from natural products ( vide infra). Molecular weight, stereocenters, rotatable bonds, hydrophobicity, and aqueous solubility are a few examples of parameters commonly included in such analyses. When applied in the context of diversity-oriented synthesis, PCA is primarily used to visualize similarities and differences within collections of compounds based on structural and physicochemical parameters, and can be leveraged in library design.
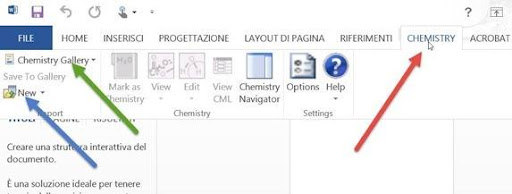
Principal component analysis (PCA) is a mathematical method for dimensionality reduction that allows for multidimensional datasets to be visualized using two- or three-dimensional plots with minimal loss of information.
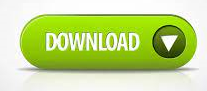